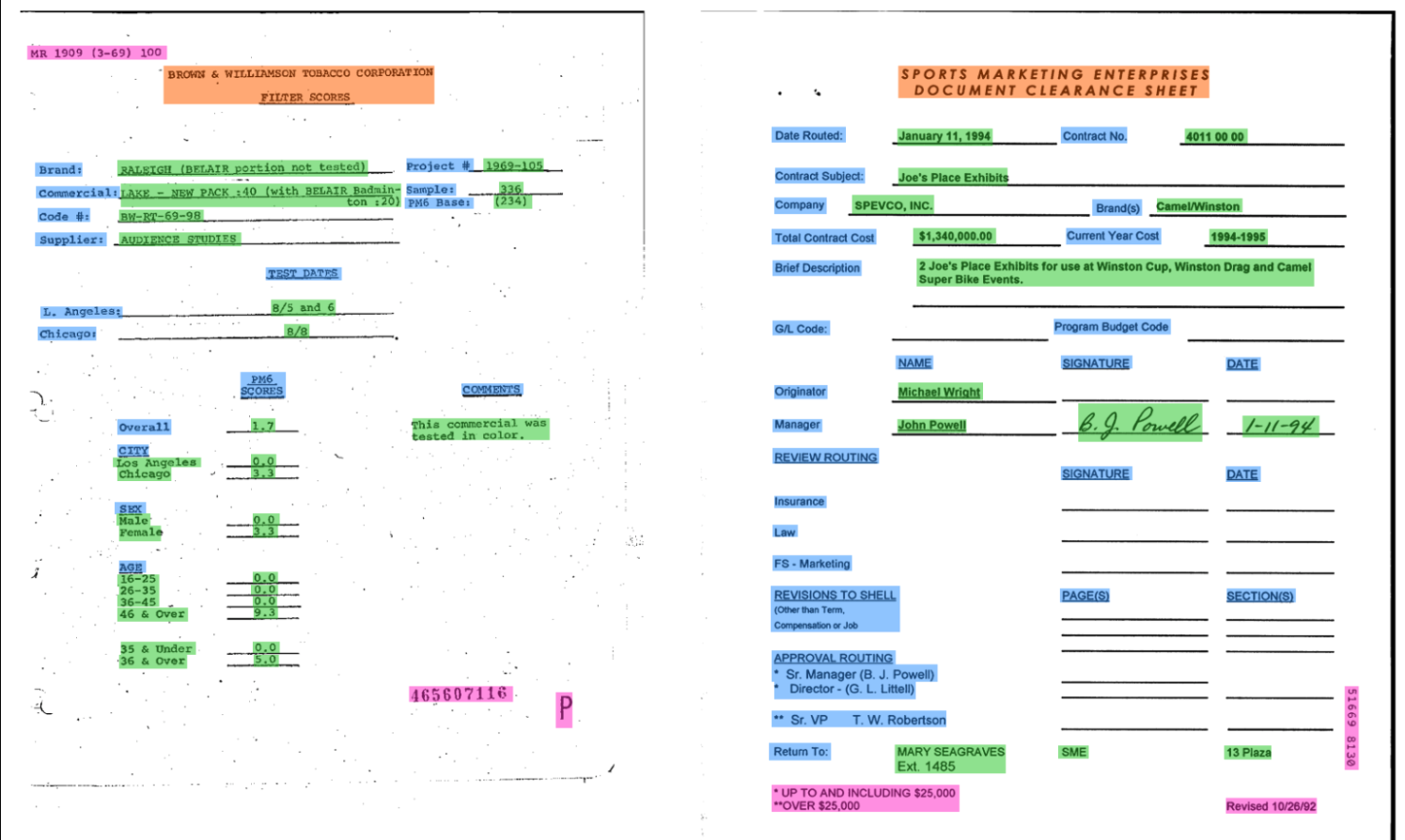
Engineering Explained: LayoutLMv3 and the Future of Document AI
Image-to-text and Document AI models have gotten much better in the past few years. The techniques that dominated industry for a long time (template matching and text-based rules) have been overpowered first by language models and now by new document models that consider not only text but visual properties of the document as well.
Visual indicators possess key context for understanding documents - what a document looks like, where certain pieces of text are on the page (bottom versus top, small versus large). The same type of document (e.g. invoice) can have a huge variety of formats, requiring a more contextually-enriched understanding of the document. Prior state-of-the-art approaches (pre-2022) perform OCR (text extraction on documents) and plug the resulting text into a language model, discarding any visual information.
LayoutLMv3 and Donut (OCR-Free Document Understanding Transformer) are two new models (released second half of 2022) that attain higher levels of document understanding by considering not just document text but the visual features of the document. LayoutLMv3 uses OCR data (text on the page including its position and size) plus a low-resolution picture of the document, from which it’s able to learn different layouts.
Donut is OCR-Free, meaning that the only input is a picture of the document itself (a document being a single page). With higher resolution (~1920p), the Donut model is able to perform text extraction from the document internally, and the extracted text is enriched with all the visual features of the document.
Either of these groundbreaking models can be trained to perform almost any document task you desire. Training both to perform the same task and using an ensembling approach (ask them both the same question as see if they agree) can make them even more powerful.