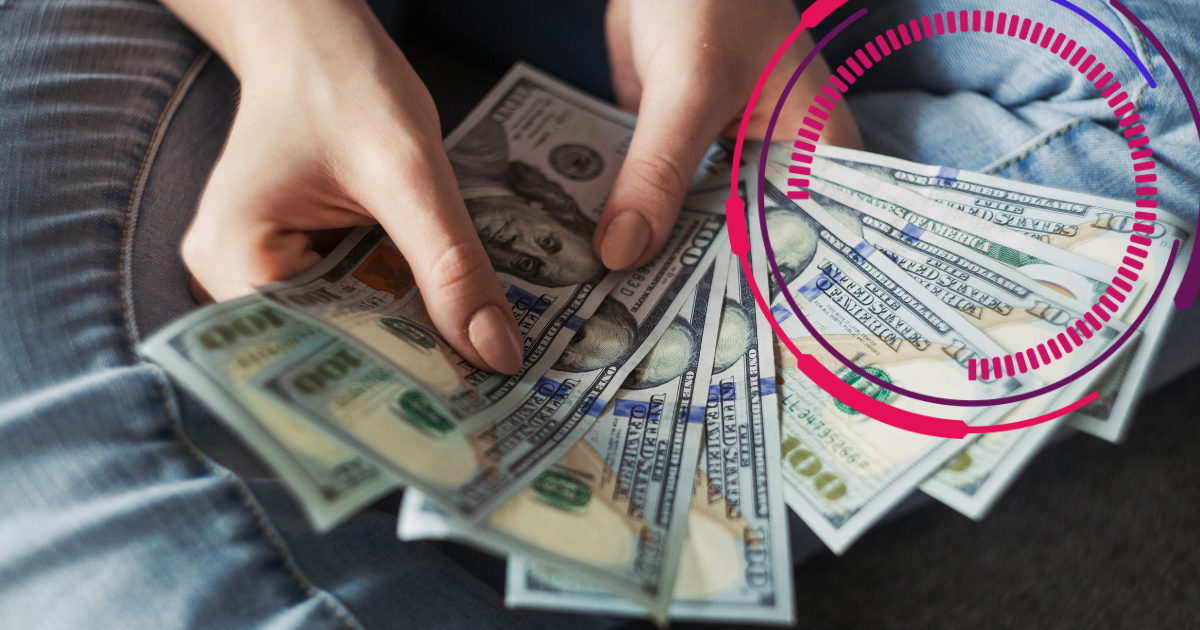
Part 2: Planning for Next Year’s AI Budget: A Strategic Guide for C-Level Executives
In the previous blog, we explored the decision-making process behind building vs. buying an AI team. It's a critical first step for any executive planning to integrate AI into their business.
Let’s start with a story that might sound familiar. I recently sat in a meeting where the CEO of a medium-sized enterprise laid out an ambitious plan to leverage AI to revolutionize their customer service operations. The room buzzed with excitement. They had read the reports, heard the success stories, and wanted to jump in with both feet. However, when it came time to discuss the budget, the mood shifted. No one knew what the real costs would be, or how to even begin. The enthusiasm fizzled, and the project was put on ice, yet another brilliant idea that failed to take off, not because it wasn’t worth pursuing, but because no one had mapped out the financial roadmap to get there.
Sound familiar? It’s a scenario playing out across boardrooms everywhere as companies grapple with AI’s promise. The potential is massive, but the planning is shaky at best. AI budgets are notoriously tricky because AI itself is unlike any other investment. You don’t just buy software and plug it in. It’s an evolving, iterative process that requires a completely different mindset and budgeting process.
The Pain Point: AI Isn't Just Another Line Item
Let’s be real. You, as a C-suite leader, have a lot on your plate. You’ve been tasked with making sure your company stays ahead of the curve, and AI is a critical part of that plan. But here’s where things get sticky. AI isn’t like investing in traditional IT projects. You can’t just allocate funds the same way you would for cloud migration or software upgrades. AI projects involve multiple rounds of experimentation, changes in strategy, and often require resources far beyond what most organizations initially anticipate.
This is where most companies get stuck, they assume that AI can be handled with the same kind of budgeting process they’ve used for years. But here’s the harsh reality: treating AI like a traditional IT expense is a recipe for failure. You need a new framework, one that accounts for the nuances, complexity, and continuous nature of AI investments.
The Contrarian View: AI Isn’t Expensive, Bad AI Planning Is
Here’s a bold claim: AI itself isn’t inherently expensive, but bad AI planning is. Let that sink in for a moment. Companies aren’t blowing through budgets because AI is prohibitively costly, they’re overspending because they’re not thinking strategically and they’re not budgeting correctly from the start.
Think about it. Companies budget for AI projects without fully understanding the underlying technology, the skill sets needed, or the ongoing operational costs. They dive in without asking key questions like: What are the business objectives? Do we have the right skill sets and data infrastructure? How will this project scale? Do we have a defensible ROI framework and calculation?
Then, halfway through, they’re hit with unexpected costs that balloon out of control. The problem isn’t the cost of AI, it’s the failure to plan for AI and manage the project effectively.
Adding to the Problem: The Cost of Missteps
Let’s look at what’s at stake. Imagine your team invests in an AI system to improve customer recommendations on your e-commerce platform. You allocate $500,000 to cover the costs of the technology, data scientists, and implementation. Six months in, you realize that your team is struggling to productionalize their proof-of-concept because they don’t have all the needed skill-sets, data quality is subpar, and you didn’t account for the additional $200,000 needed to clean, label, and integrate your data. Next, you discover that your initial tech stack isn’t scalable, and the cloud infrastructure you thought would handle the workload is costing you double what you anticipated.
These are common, and yet avoidable, missteps that can sink your project. And what’s worse? You risk losing credibility internally and externally. The CEO is frustrated. The CFO wants to know why the project is bleeding cash. The board and investors are questioning progress and expecting measurable outcomes. Your AI initiative, which should have been a game changer, is now seen as a costly experiment gone wrong. And, once this happens, you’re in a vicious cycle where your future AI projects are met with skepticism while your competitors are moving ahead.
A Roadmap for AI Budgeting Success
So how do you avoid these budgeting, planning and execution pitfalls? By taking a strategic and structured approach to AI budgeting. Here’s a roadmap that will help ensure your AI budget is set up to deliver success next year:
1. Align AI with Business Objectives and Secure Stakeholder Buy-in
Before a single dollar is spent, identify exactly what business outcome you’re trying to achieve. AI for the sake of AI won’t get you anywhere. Are you aiming to reduce operational costs, increase revenue through personalization, or drive efficiencies through automation? Define the business impact first because AI is just the enabler. Additionally, ensure you have buy-in from key stakeholders across departments, such as leadership, IT, and operations. Without their endorsement and collaboration, AI projects risk encountering internal resistance or misalignment with business processes. If you’re unclear what AI can do to transform your business, hire an expert to help you and your team get educated and understand where the opportunities may be in your business.
2. Invest in the Right Expertise
AI isn’t plug-and-play. It requires data scientists, machine learning engineers, software architects, and subject matter experts. Underestimating the need for specialized talent is a common mistake. Budget not just for initial hires but also for ongoing training, as AI technologies and methodologies evolve rapidly. If you’re trying to decide whether you should hire an external consultant or build an internal AI team, check out our recent blog on Building Vs. Buying a team.
3. Audit Your Data
According to studies conducted by IBM and Gartner, most companies don’t realize that 80% of the effort in an AI project revolves around data. If your data isn’t clean, organized, and relevant, your AI model will underperform. A thorough data audit at the outset can save you a lot down the road. Include data cleaning, labeling, and compliance costs in your budget upfront.
4. Plan for Iteration and Experimentation
AI projects are not a one-and-done affair. Machine learning models improve over time through iteration and conversely get worse over time without proper maintenance. That means you need to budget for research and development, testing, and model refinement. Flexibility is key so allocate a portion of your budget specifically for these ongoing experiments and adjustments.
5. Factor in Infrastructure Costs
AI is resource-intensive. Whether you’re using cloud-based infrastructure or investing in on-premise hardware, don’t underestimate the cost of computing power, storage, and bandwidth. Make sure your infrastructure budget accounts for scalability. What works for a proof of concept won’t necessarily handle an enterprise-wide deployment.
6. Plan for Integration, Deployment and Change Management
Budget for the cost of integrating AI systems into existing workflows and technologies. This often includes change management efforts such as training your teams on how to use the new tools effectively, creating mechanisms for feedback collection, and ensuring the AI system can coexist, or intentionally not, with legacy systems. The proponderance of the challenges with AI implementations aren’t about the technology but the people involved. This stage is often where projects face delays if not properly planned for.
7. Monitoring and Post-Deployment Support
AI doesn’t stop at deployment. You need to budget for continuous monitoring, model retraining, and system updates. AI models degrade over time if left unchecked, and regular performance evaluations are crucial to maintaining their accuracy and relevance. A common mistake people make is to assume AI projects aren’t beholden to all the rigors of traditional software deployment, they should be.
8. Don’t Forget the Contingency
Finally, build a contingency reserve into your budget—typically 10-20%. AI projects are exploratory by nature, and you’ll want financial flexibility to handle unforeseen challenges or shifts in strategy.
Conclusion: AI Budgeting Is About Long-Term Value, Not Short-Term Savings
AI has the potential to transform your business, but only if you approach it with the right mindset and financial planning. This isn’t about finding ways to cut costs in the short term, it’s about investing in a technology that, when planned and executed correctly, will deliver exponential value in the long run.
The budget cycle that we are entering into right now is very consequential as it relates to AI. Some companies will take the approach of staying on the sidelines and end up playing catch-up or be left behind. Others will underinvest in AI and/or try to bluster their way through and undoubtedly experience setbacks. This will reduce support for future AI budgets, which will put these companies at a long-term competitive disadvantage.
The likely winners in the new era of AI will have leaders who have the confidence to be vulnerable to acknowledge that AI is new and different and they don’t know all that there is to know. This humility, along with seeking outside expertise and developing a learning mentality, will be key ingredients to success.
The companies that succeed in AI aren’t necessarily the ones that throw the most money at it, they’re the ones that budget strategically, understand the ongoing nature of AI development, execute well, and focus on aligning their initiatives with long-term business goals. In 2025, make sure you’re one of them.
After this, there are two more important topics we’ll cover in the series:
- How to Write and Manage an AI RFP – Discover how to build an effective RFP that will help you clarify your AI use cases and find the right vendors.
- Finding the Right AI Partner – We’ll conclude the series by discussing how to evaluate and choose the best AI partner for your organization.
Ready to start planning your AI budget for next year? Let’s talk strategy.